Abstract
At the 2023 NSF NRT Annual Meeting, I presented a poster on native language processing impacts on human language technology. My collaborators and I used EEG to evaluate neural processing differences when native Spanish listeners hear matched versus mismatched native accent variants. We found evidence that unfamiliar variants incur cognitive costs. As language ID and speech recognition systems currently struggle with non-native speech, I’m integrating psychological and computational linguistics techniques to explore improvements. This project synthesizes varied disciplines - from psycholinguistics to natural language processing - to address inclusion gaps in speech technology across accent variants. Enhancing system robustness to diverse forms of speech is essential as virtual assistants and other AI applications continue permeating everyday life.
Date
Oct 30, 2023 9:00 AM — 10:00 AM
Location
Arizona State University
1151 S Forest Ave, Tempe, Arizona
Click on the Slides button above to view the built-in slides feature.
Slides can be added in a few ways:
- Create slides using Hugo Blox Builder’s Slides feature and link using
slides
parameter in the front matter of the talk file - Upload an existing slide deck to
static/
and link using url_slides
parameter in the front matter of the talk file - Embed your slides (e.g. Google Slides) or presentation video on this page using shortcodes.
Further event details, including page elements such as image galleries, can be added to the body of this page.
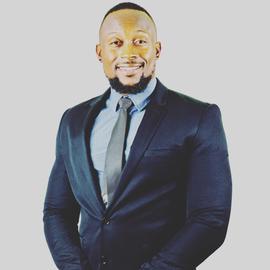
Ph.D. Student in Informatics
My research interests include low-resource multilingual NLP, linguistics, adversarial machine learning and mis/disinformation generation/detection. My Ph.D. thesis is in the area of applying artificial intelligence for cybersecurity and social good, with a focus on low-resource multilingual natural language processing. More specifically, I develop NLP techniques to promote cybersecurity, combat mis/disinformation, and enable AI accessibility for non-English languages and underserved populations. This involves creating novel models and techniques for tasks like multilingual and crosslingual text classification, machine translation, text generation, and adversarial attacks in limited training data settings. My goal is to democratize state-of-the-art AI capabilities by extending them beyond high-resource languages like English into the long tail of lower-resourced languages worldwide. By innovating robust learning approaches from scarce linguistic data, this research aims to open promising directions where AI can have dual benefits strengthening security, integrity and social welfare across diverse global locales.